The financial landscape is undergoing a seismic shift with the advent of robo-trading, where artificial intelligence (AI) is not just an aid but a dominant force in market transactions. This article delves into the intricacies of robo-trading, examining its evolution, the technologies at its core, and the profound impact it has on trading strategies, market dynamics, and regulatory frameworks. We explore the ethical debates it sparks and the future it heralds for financial markets, as AI continues to redefine the boundaries of what's possible in trading.
Key Takeaways
- Robo-trading is revolutionizing financial markets with advanced algorithms and machine learning techniques, outpacing traditional human decision-making.
- AI-driven trading strategies are enhancing efficiency and customization, but they also introduce new complexities in risk management.
- The integration of AI in trading is altering market behavior, potentially improving market efficiency but also raising concerns about high-frequency trading practices.
- Ethical and regulatory challenges abound as the fairness and transparency of AI trading systems come under scrutiny, with ongoing debates about the need for human oversight.
- As financial technologies evolve, the future landscape of markets will likely see deeper integration of AI, necessitating preparedness for its unforeseen consequences.
Understanding Robo-Trading
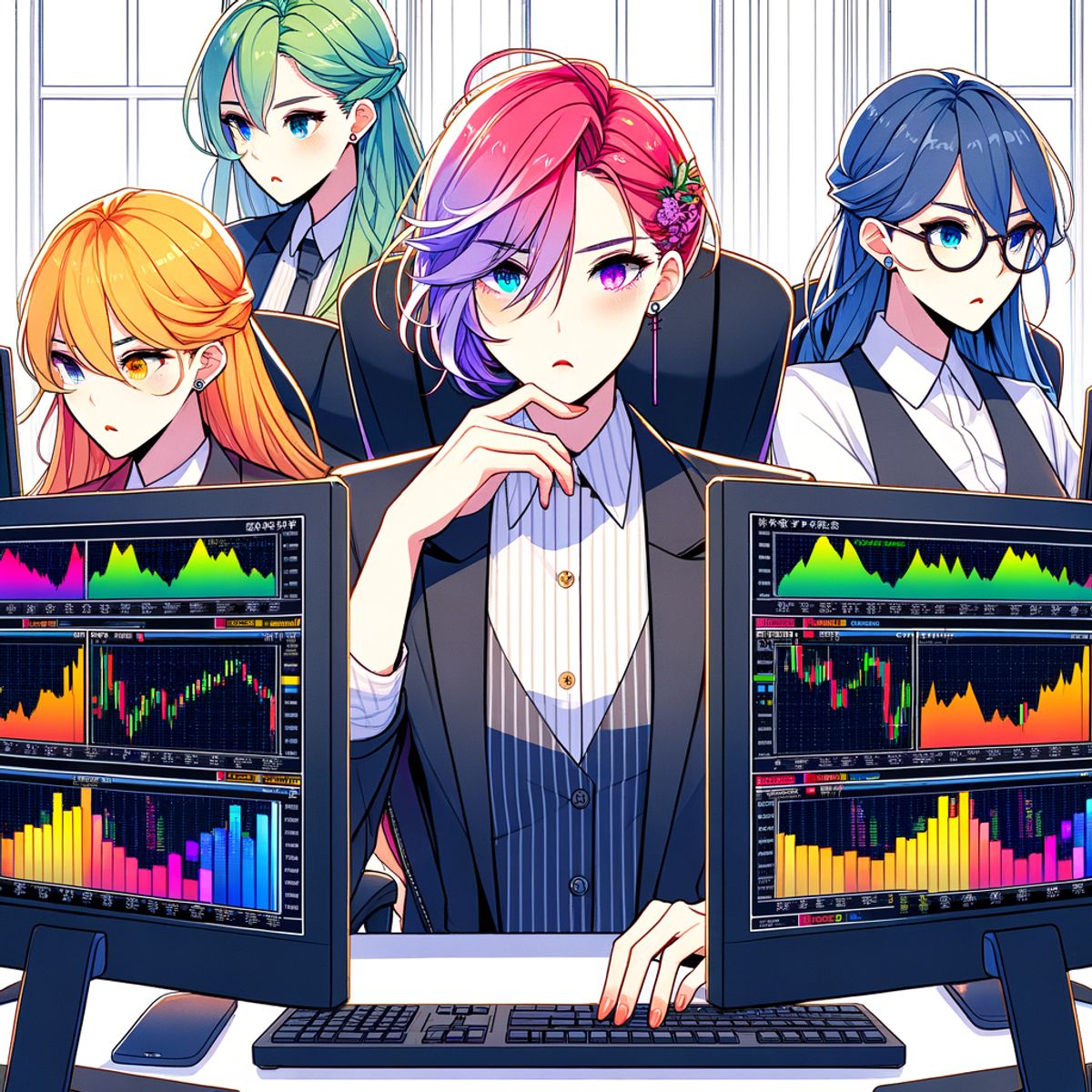
Defining Robo-Trading and Its Capabilities
Robo-trading, also known as algorithmic trading, refers to the use of computer algorithms to execute trades in financial markets with speed and efficiency that surpass human capabilities. These systems can analyze vast amounts of market data, execute trades at optimal prices, and exploit trading opportunities in a fraction of a second.
- Speed: Trades executed in milliseconds
- Precision: Reduced risk of manual errors
- Analysis: Real-time processing of large datasets
- Consistency: Unaffected by human emotions
Robo-trading has revolutionized the way markets operate, offering a level of precision and speed that is reshaping the landscape of financial trading.
The capabilities of robo-trading systems extend beyond mere execution of trades. They are also capable of monitoring market conditions, predicting price movements, and managing risks, all while adhering to a predefined set of trading rules. This automation not only increases the efficiency of trading operations but also opens up new strategies that were previously impossible or impractical for human traders.
Historical Development of Automated Trading Systems
The evolution of automated trading systems has been a game-changer in the world of finance, particularly in the forex market. Forex expert advisors, a type of automated trading software, have revolutionized the way currency trading is conducted. These systems have evolved from simple automated programs to complex algorithms capable of making decisions based on market analysis and trends.
The inception of electronic trading platforms in the 1970s marked the beginning of a new era in trading, paving the way for the development of sophisticated automated systems.
The timeline below highlights key milestones in the historical development of automated trading systems:
- 1970s: Introduction of electronic trading platforms.
- 1980s: Development of basic automated trading systems.
- 1990s: Emergence of retail forex trading and the first forex expert advisors.
- 2000s: Advancements in computational power and data analysis techniques.
- 2010s: Integration of machine learning and artificial intelligence in trading algorithms.
As these systems became more advanced, they started to include features such as historical data analysis, real-time market scanning, and predictive modeling, which have significantly increased their predictive accuracy and reliability.
Key Technologies Powering Robo-Trading
The technological backbone of robo-trading is a fusion of sophisticated software and advanced computational frameworks. Machine Learning (ML) and Artificial Intelligence (AI) are at the forefront, enabling systems to learn from data, identify patterns, and make decisions with minimal human intervention.
- Data Analytics: Crunching vast amounts of market data to uncover insights.
- Natural Language Processing (NLP): Interpreting news and social media for sentiment analysis.
- Cloud Computing: Providing the necessary computational power and storage.
- Blockchain: Ensuring secure and transparent transaction records.
The integration of these technologies has not only automated the trading process but also enhanced the ability to predict market movements and execute trades at an unprecedented speed and volume. The synergy between high-speed data processing and real-time analytics has given rise to a new era of market strategies that were previously impossible.
AI's Impact on Trading Strategies
Algorithmic Trading vs. Human Decision-Making
The financial markets have witnessed a paradigm shift with the advent of algorithmic trading, which stands in stark contrast to traditional human decision-making. Algorithmic trading systems can process vast amounts of data and execute trades at speeds unattainable by humans, capitalizing on market inefficiencies in mere fractions of a second.
- Human traders base decisions on a combination of analysis, intuition, and emotional responses.
- Algorithms operate on predefined rules and can be backtested on historical data to refine strategies.
- Human traders are constrained by physical and cognitive limitations, such as the need for sleep and susceptibility to stress.
The relentless efficiency of algorithms has redefined the landscape of trading, challenging the role of human intuition and experience in a market increasingly dominated by machines.
While algorithms excel in consistency and speed, they lack the nuanced understanding of market sentiment that seasoned traders possess. This dichotomy raises important questions about the future role of human traders and the potential for a symbiotic relationship between man and machine in the financial arena.
Customization and Optimization of Trading Algorithms
The advent of AI for trading has revolutionized the way financial markets operate, with customization and optimization at the forefront of this transformation. Robo-trading algorithms can now be tailored to specific investment strategies, allowing for a level of precision and efficiency previously unattainable.
The ability to customize trading algorithms means that AI systems can learn and adapt to new market conditions in real-time, constantly refining strategies to maximize returns.
Optimization techniques vary, but they often include backtesting against historical data, forward testing in simulated environments, and continuous learning through machine learning models. Below is a list of common optimization methods used in AI for trading:
- Backtesting with historical market data
- Forward testing with paper trading
- Machine learning for pattern recognition and prediction
- Genetic algorithms to evolve strategy parameters
- Sentiment analysis for gauging market mood
Each method contributes to creating a robust trading system that can navigate the complexities of the financial markets with a high degree of autonomy.
Risk Management in an AI-Dominated Environment
In an AI-dominated trading environment, risk management strategies have evolved significantly. Robo-trading systems, particularly the best ea using ai, have become adept at identifying and mitigating potential risks. These systems can process vast amounts of data to recognize patterns that may indicate market downturns or volatile conditions, allowing for preemptive action.
The integration of advanced analytics and machine learning techniques has enabled the development of more sophisticated risk management tools. These tools can adapt to changing market conditions in real-time, providing traders with a dynamic shield against unforeseen market movements.
The best ea using ai not only automates trades but also incorporates features designed to minimize losses. For instance, they can automatically adjust stop-loss orders based on market sentiment analysis or implement hedging strategies to protect investment portfolios. The table below illustrates some of the key functionalities that enhance risk management in AI-powered trading systems:
Functionality | Description |
---|---|
Stop-Loss Adjustment | Automatically modifies stop-loss orders to lock in profits and limit losses. |
Hedging Strategies | Employs various techniques to offset potential losses in one position by gains in another. |
Volatility Assessment | Analyzes market volatility to adjust trading strategies accordingly. |
Portfolio Diversification | Ensures a balanced investment spread to mitigate risk exposure. |
As the landscape of financial markets continues to shift towards greater AI involvement, traders and institutions must remain vigilant in updating and refining their risk management protocols. The ea using ai represents a significant step forward, but it is essential to maintain a balance between reliance on technology and human expertise.
Market Dynamics in the Age of AI
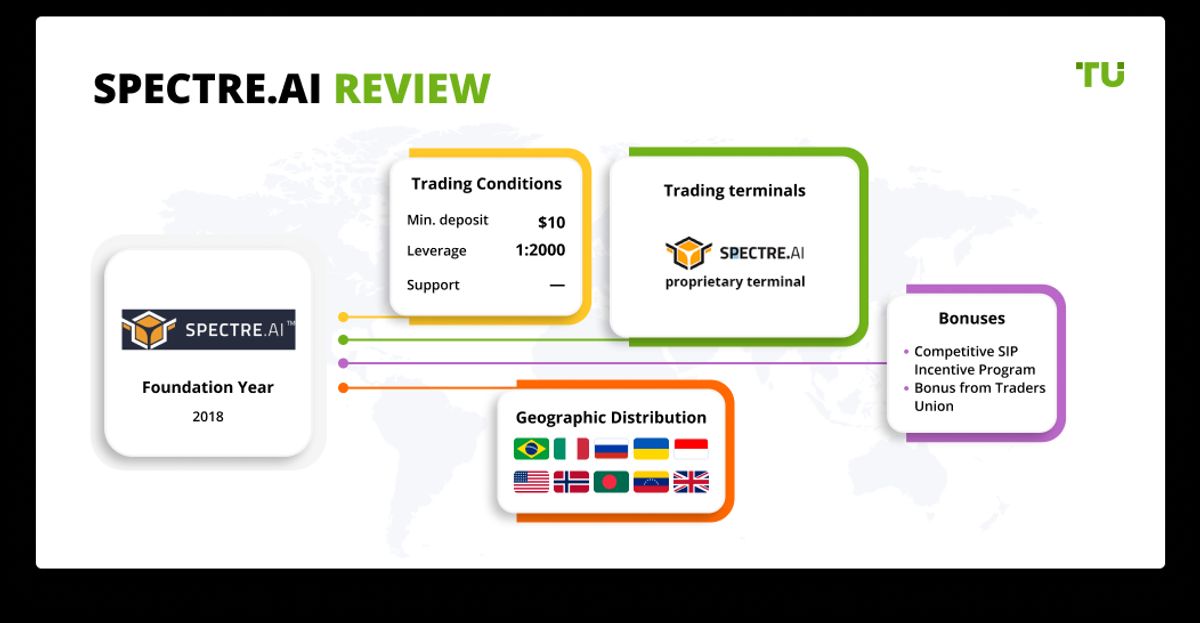
How AI is Changing Market Behavior
The integration of Artificial Intelligence in trading has led to significant shifts in market behavior. AI's ability to analyze vast datasets has resulted in markets that are more reactive to real-time information. This responsiveness has both streamlined and complicated the trading landscape.
- AI-driven strategies can detect subtle patterns and trends that are imperceptible to human traders.
- The speed of AI systems allows for rapid execution of trades, often capitalizing on fleeting market opportunities.
- AI's predictive analytics contribute to anticipatory movements in the market, sometimes leading to self-fulfilling prophecies.
The convergence of AI with market data has not only accelerated the pace of trading but also raised the bar for what is considered informed decision-making in financial markets.
The implications of these changes are profound, with AI reshaping the very fabric of market dynamics. As AI continues to evolve, its influence on market behavior is expected to deepen, potentially leading to new forms of market strategies and participant interactions.
The Influence of AI on Market Efficiency
The integration of AI in trading has sparked a significant debate regarding its impact on market efficiency. AI's ability to process vast amounts of data and execute trades at superhuman speeds is argued to enhance market liquidity and price discovery. However, this efficiency comes with caveats; the speed and complexity of AI-driven trades can sometimes lead to market volatility and flash crashes.
- AI-driven algorithms can detect price inefficiencies faster than human traders.
- These algorithms contribute to tighter bid-ask spreads, benefiting all market participants.
- However, the opacity of algorithmic strategies may obscure true market sentiment.
The question remains whether the benefits of increased efficiency outweigh the potential for systemic risks introduced by AI. This balance is critical in ensuring that markets remain fair and orderly in the age of automation.
AI's Role in High-Frequency Trading
High-frequency trading (HFT) represents the pinnacle of AI's integration into financial markets, where algorithms execute thousands of orders in fractions of a second. The speed and efficiency of AI systems in HFT are unmatched by human traders, leading to a landscape where microseconds can mean the difference between profit and loss.
- AI algorithms can analyze vast datasets to identify fleeting market inefficiencies.
- They execute trades at speeds no human can match, often reacting to market events before they fully unfold.
- The strategies employed by HFT AI can adapt in real-time to changing market conditions, constantly evolving to maintain a competitive edge.
In the realm of HFT, AI's ability to process and act on complex market data at lightning speeds has not only transformed trading strategies but also raised questions about market fairness and the potential for systemic risks.
The relentless pursuit of speed has led to technological innovations such as microwave transmission towers and advanced order types. These developments have further entrenched AI's dominance in HFT, making it an indispensable tool for firms looking to capitalize on the smallest price discrepancies.
Ethical and Regulatory Considerations
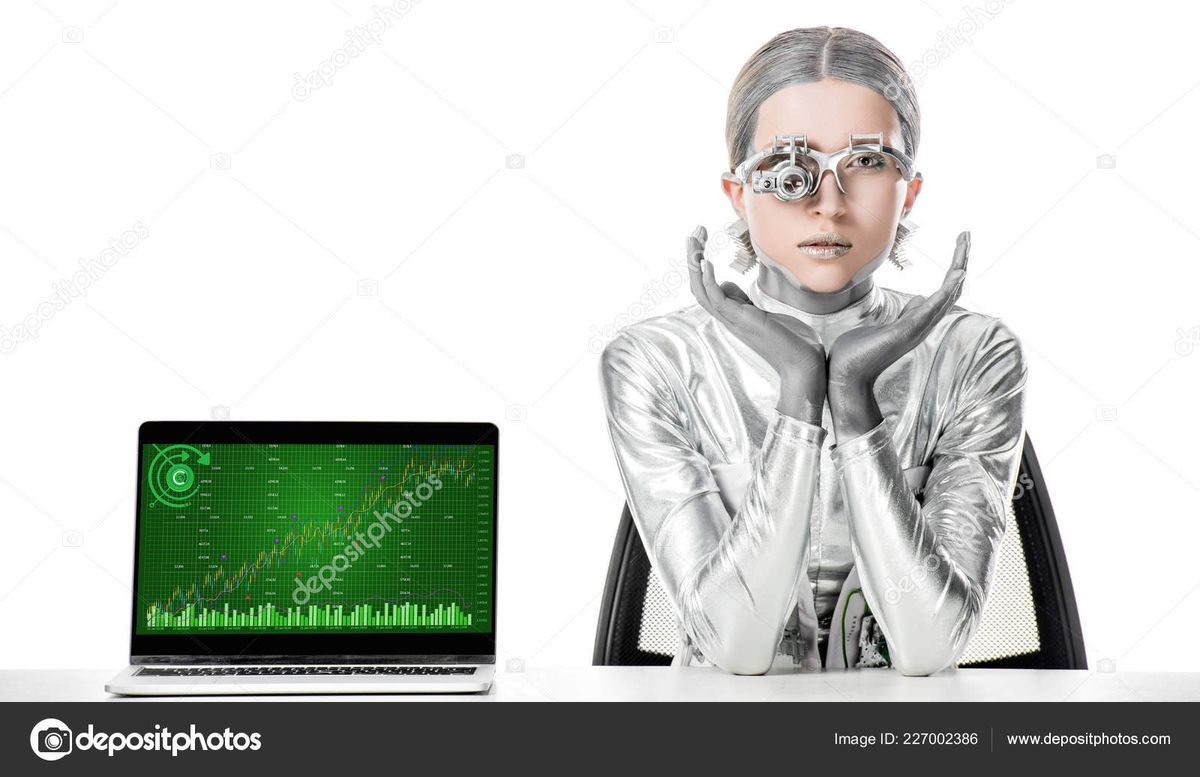
The Debate Over Fairness and Transparency
The integration of AI in trading has sparked a significant debate over the fairness and transparency of financial markets. Concerns center on the potential for AI to create information asymmetries, where algorithmic traders may have an unfair advantage over human traders. This advantage stems from their ability to process and act on vast amounts of data at speeds unattainable by humans.
The question of whether this constitutes an unfair playing field is at the heart of the debate, with many arguing that it undermines the principle of equal access to information that is fundamental to free markets.
The following points highlight key concerns in this debate:
- The opacity of algorithmic decision-making processes.
- The challenge of ensuring compliance with existing financial regulations.
- The difficulty in monitoring and understanding AI-driven market manipulation tactics.
Regulators are grappling with these issues, trying to balance the benefits of AI-driven efficiency with the need for a fair and transparent market environment.
Regulatory Challenges in a Rapidly Evolving Field
As robo-trading technologies advance at a breakneck pace, regulators are faced with the daunting task of keeping up. The dynamic nature of AI systems complicates the creation of a stable regulatory framework, often leading to a lag between innovation and oversight.
Regulatory bodies must consider a range of factors:
- Ensuring transparency in AI decision-making processes
- Protecting investors from algorithmic malfunctions
- Establishing accountability for autonomous trading actions
The challenge lies in balancing the need for innovation-friendly policies with the protection of market integrity and investor interests.
The rapid evolution of AI in trading also raises questions about the adequacy of existing financial regulations. Are they flexible enough to accommodate new technologies, or will they stifle growth? This ongoing debate is critical as it will shape the future of financial markets and the role AI plays within them.
The Future of Human Oversight in AI Trading
As we venture further into the era of AI-driven financial markets, the role of human oversight becomes a complex puzzle. The balance between automated efficiency and human judgment is delicate, and the financial industry must navigate this with care. The integration of AI in trading has raised questions about the necessity and form of human intervention.
- Ensuring compliance with evolving regulations
- Interpreting AI decisions in the context of market anomalies
- Maintaining ethical standards in automated trading
- Providing a safeguard against systemic risks
The challenge lies not only in establishing effective oversight mechanisms but also in continuously adapting them to keep pace with AI advancements. Human oversight must evolve to be more about strategic decision-making and less about manual intervention, focusing on the oversight of AI systems rather than individual trades.
The future of human oversight in AI trading is not about replacing one with the other but finding the optimal synergy that leverages the strengths of both. As AI systems become more autonomous, the human role will likely shift towards governance, ethical considerations, and crisis management, ensuring that the digital heartbeat of the markets aligns with human values and societal norms.
The Future Landscape of Financial Markets
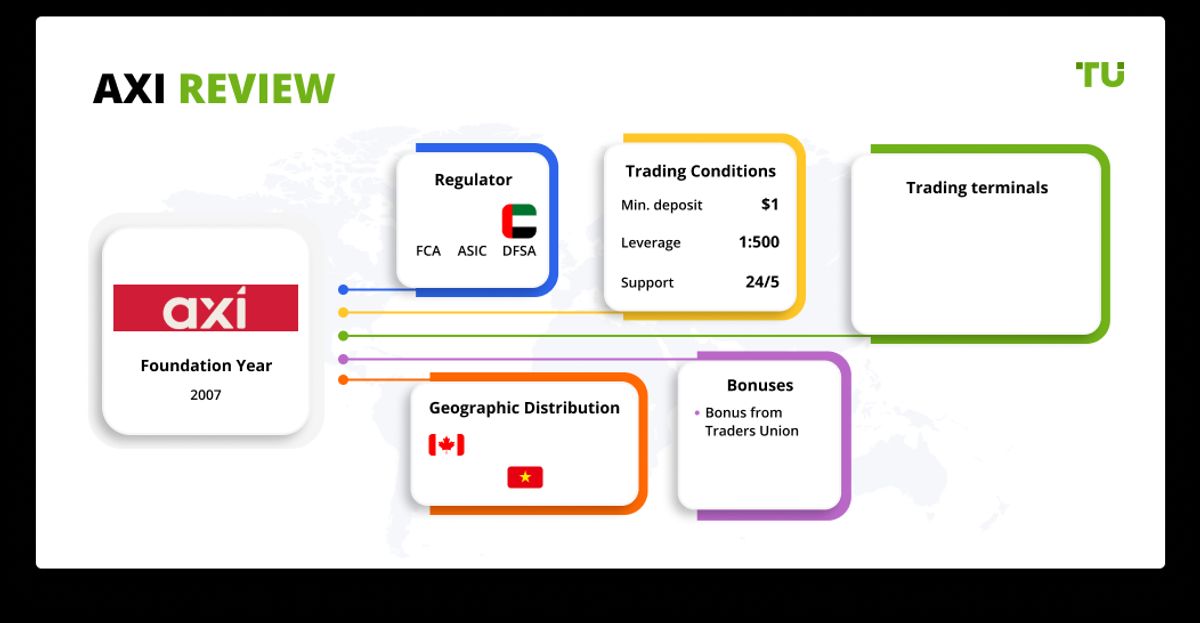
Predicting the Next Evolution of Robo-Trading
As we stand on the cusp of new advancements in financial technology, the next evolution of robo-trading seems poised to further disrupt the status quo. The integration of deeper learning algorithms and quantum computing could redefine the boundaries of trading efficiency and speed, leading to unprecedented levels of market analysis and execution capabilities.
- Enhanced predictive analytics for market trends
- Real-time risk assessment and management
- Greater adaptability to market volatility
- Personalized trading strategies for individual investors
The synergy between AI and emerging technologies is expected to create a more intuitive and responsive trading ecosystem, where decisions are made in fractions of a second and are continuously refined through iterative learning.
The potential for AI to autonomously develop and evolve trading strategies could lead to a landscape where human traders and traditional financial analysts find their roles significantly transformed. The challenge for the industry will be to harness these changes in a way that benefits all market participants and maintains a stable financial environment.
Integrating AI with Emerging Financial Technologies
The integration of AI with emerging financial technologies is poised to create a new frontier in the financial markets. Blockchain technology, for instance, offers a decentralized ledger that can enhance the transparency and security of AI-driven transactions. Meanwhile, the advent of cryptocurrencies has opened up novel trading strategies that AI systems are uniquely equipped to exploit.
- Blockchain: Enhances security and transparency in AI transactions.
- Cryptocurrencies: Offers new avenues for AI trading strategies.
- Smart Contracts: Automates and enforces contract terms with precision.
- Decentralized Finance (DeFi): Expands AI's role in autonomous financial services.
The synergy between AI and these technologies promises to streamline operations, reduce costs, and open up new opportunities for innovation. As AI algorithms become more sophisticated, they will be able to interact with these technologies in increasingly complex ways, potentially reshaping the landscape of financial services.
The challenge lies in ensuring that these integrations are done in a way that maintains market integrity and protects investors. As AI becomes more entrenched in these emerging domains, it will be crucial to monitor its influence and ensure that it contributes positively to the evolution of the financial ecosystem.
Preparing for the Unforeseen Consequences of AI Trading
As the financial markets continue to intertwine with advanced AI technologies, stakeholders must remain vigilant and proactive in preparing for the unforeseen consequences of robo-trading. The unpredictable nature of AI-driven markets necessitates a robust framework for risk assessment and management.
To navigate these uncharted waters, market participants should consider the following steps:
- Continuously monitor AI systems for aberrant behavior or potential malfunctions.
- Develop contingency plans for AI-induced market disruptions.
- Advocate for and participate in the creation of industry-wide standards and protocols.
- Invest in ongoing education and training to understand AI's evolving role in financial markets.
While we cannot predict every outcome, the commitment to adaptability and resilience will be paramount in safeguarding the integrity of financial markets in the face of AI's advancement.
Conclusion: Embracing the New Era of AI in Finance
The ascent of robo-trading marks a transformative era in the financial markets. As artificial intelligence continues to evolve, its dominance is not just a trend but a paradigm shift in how trading is conducted. The integration of AI in trading strategies has demonstrated enhanced efficiency, speed, and analytical capabilities, outperforming traditional methods in various aspects. However, this rise also brings forth challenges such as regulatory concerns, ethical considerations, and the need for transparency. As we stand at the cusp of this technological revolution, it is imperative for industry participants, regulators, and individuals to adapt, innovate, and establish frameworks that harness the potential of AI while safeguarding the integrity of the markets. The future of finance is inextricably linked with AI, and the journey ahead promises to be as exciting as it is unpredictable.
Frequently Asked Questions
What is robo-trading and how does it work?
Robo-trading, also known as automated or algorithmic trading, involves the use of computer programs and artificial intelligence to execute trades in financial markets based on predefined criteria, without human intervention. These systems analyze market data, make predictions, and execute trades at high speeds and volumes, often outperforming human traders.
How have automated trading systems evolved over time?
Automated trading systems have evolved from simple rule-based programs to sophisticated AI-driven platforms that can learn and adapt to market conditions. The development of machine learning, big data analytics, and high-speed computing has significantly enhanced the capabilities of these systems since their inception.
In what ways do AI trading strategies differ from human decision-making?
AI trading strategies are based on algorithms that can process vast amounts of data and execute trades based on statistical probabilities, without being influenced by emotions or biases. In contrast, human decision-making in trading often involves psychological factors and may not consistently handle the same volume or speed of information as AI.
What role does AI play in managing risk in financial trading?
AI plays a crucial role in risk management by providing tools for real-time monitoring, predictive analytics for market trends, and the ability to quickly adjust strategies in response to changing market conditions. This helps in mitigating potential losses and optimizing the risk-reward ratio in trading portfolios.
How is AI influencing the efficiency of financial markets?
AI contributes to market efficiency by enabling faster and more accurate processing of information, which helps in the discovery of fair prices. However, it also raises concerns about market manipulation and the creation of artificial market conditions due to the speed and volume of trades executed by AI systems.
What are the main regulatory challenges associated with robo-trading?
Regulatory challenges include ensuring fairness and transparency in markets dominated by AI, preventing market abuse, and keeping up with the rapid pace of technological advancements. Regulators are also tasked with understanding complex AI systems and devising appropriate frameworks to govern their use in trading.
Social Plugin